Facial Recognition Technology in India
- Rishab Krishnamurthy
- Oct 1, 2021
- 17 min read
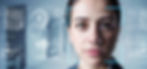
Executive Summary
This article aims to explain what facial recognition technology is and analyse issues in its implementation. The issues covered cover both the legal and technical aspects of implementing this technology. It goes over legislation and judgements relevant to this topic and also addresses algorithmic biases and how they develop. Finally, the article covers recommendations that address some of the concerns surrounding this technology.
Keywords: Facial Recognition, Privacy, Algorithmic Bias, Personal Data Protection Bill.
Introduction
On the 22nd of July 2020, the National Crime Records Bureau (NCRB) put out a Request For Proposal (RFP) [1] concerning the creation of an Automatic Facial Recognition System (AFRS) in India. The stated purpose of the system as per the RFP is to ensure the “Availability of relevant and timely information” to “improve outcomes in Criminal identification and verification by facilitating easy recording, analysis, retrieval and sharing of information between different organisations.”
The RFP then goes on to describe the requirements of the AFRS that the NCRB is looking for. This move by the NCRB has raised concerns for digital rights activists due to several factors such as the inaccuracy of facial recognition technology, infringement of citizens' right to privacy, etc.
This paper endeavours to explain what facial recognition technologies are, the state of facial recognition worldwide and in India, and examine arguments surrounding their implementation in state infrastructure.
A Primer on Facial Recognition Technologies
For this piece to make sense, we first need to understand what Facial Recognition Technologies (hereinafter FRTs) are, how they work, where they are used, and how their performance is measured. This will allow us to understand the technical aspects of FRTs before diving into other areas.
What are FRTs?
FRTs can be broadly classified based on the functions they perform:
Whether the technology can detect a face.
Identification of features that the detected face possesses.
Identification of the person who possesses the detected face.
Face Detection Technology
The process of face detection (i.e., technologies that fall under category (1)) does not determine the individual's identity or what facial features (i.e., race, gender, and other features) they possess. The technology can only detect if a face exists within a given image. This is usually the first step in any kind of facial analysis since the subsequent analysis of features or finding the person's identity is predicated on the detection of a face.
Any facial detection technology has two possible errors [2]:
False Negatives: Not recognising a face.
False Positives: Recognising an object that is not a face as a face.
Feature Detection
Feature detection technologies identify a particular feature of an individual such as race, sex, emotional state, etc., but not their identity. Feature detection can be further subdivided into two categories:
Facial Attribute Classification [3]:
This is the task of classifying various attributes of a facial image - e.g., whether someone has a beard, is wearing a hat, and so on.
This is a challenging problem because faces can vary dramatically from one person to the other, and can be viewed under a variety of different poses, occlusions and lighting conditions.
Facial Expression Classification:
Classifying the face based on the expression it presents.
This too is a complex problem due to the wide variety of faces, and also because of the variety of expressions that the human face can make.
Identification
The final type of facial recognition technology is a technology that can identify a person using their face. This technology first detects a face, classifies a face based on the attributes, and finally uses this information to identify the person.
This type of technology is divided into two sub-categories:
Face Verification (one type of face recognition):
It attempts to determine whether an image shows a particular person.
When presented with the face, the system determines whether the face belongs to someone that the system knows.
This is used, for example, to unlock some phones, as a security mechanism etc.
Face Identification:
This system aims to understand whose face is the one that has been detected.
Once the face has been detected, it then proceeds to match the information to someone whose image information it already has.
Essentially, the software aims to answer the question: “Whose face is this?”
Facial Recognition Process
Now that we have established the different types of FRTs, we will now proceed to understand how they work. The process of facial recognition involves the use of the aforementioned technologies. The steps are:
Image capture and detection:
A face is photographed, which has a twofold purpose:
Building a repository of faces for the technology to compare to.
The photograph is compared with the repository in order to identify the individual.
Next, the face is detected.
If the face is not detected, then the photograph needs to be retaken.
Enrolment into the system:
Recognition of a face is contingent on the prior registration of the face in the database.
The process by which an individual's face is stored in the system is called enrolment.
Digital representation of the face:
The face capture process transforms the analogue information (the face) into a set of digital information (data) that is based on the person's facial features.
This data is called a faceprint.
In the same way that thumbprints are unique, each person has their faceprint.
Comparison:
Comparison of the faceprint with the database.
The comparisons generate match scores to identify the closest possible match to the given faceprint.
Decision:
Output is given. This could be the detection of a face, feature or full facial identification.
General uses of FRTs
FRTs are seeing more use today. For example, we see their use in:
Banks: Some financial institutions use FRTs as an added layer of authentication.
Consumer products: Some products use FRTs to grant access to the user.
Housing: Individual homeowners can install camera systems that use FRTs in products like smart doorbells.
Workplaces: Employers can use FRTs as access control measures i.e., restricting access to certain employees only.
Other areas of use are: Educational Institutions, Policing, Events etc.
With this foundation established, we will now examine India's use of FRTs.
India and FRTs
What is the status of FRTs in India?
We see the use of the FRTs in the following states. The list is not a complete list of FRTs in India:
Telangana:
As per the Internet Freedom Foundations Project Panoptic [5] (which aims to bring transparency and accountability to the relevant government stakeholders involved in the deployment and implementation of FRT projects in India) Telangana has the largest number of FRT projects being rolled out.
Project Panoptic lists out six projects that are present in Telangana.
The ones that stand out are the TSCOP [6] and the Crime and Criminal Network and Tracking System (CCTNS)[7].
TSCOP aims to empower front-line police officers with actionable intelligence to increase their efficiency.
The CCTNS provides services such as “Petition Management, FIR Management, Investigation Management, Courts and Prosecution, Station House Management, Higher Officers Module, Police Messaging System, Enterprise Search, Online Monthly Crime Review, and Criminal Intelligence System.”
Maharashtra:
The Maharashtra Police have begun rolling out the Automated Multi-Modal Biometric Identification System (AMBIS) [8].
Officials said that AMBIS was designed to identify suspects at the click of a mouse and provide information about criminal elements to other police forces, be it within the country or abroad.
The system integrates with CCTV systems in Maharashtra to apply FRT to CCTV footage.
Gujarat:
The Surat police have been provided FRT by the NEC.
The NEC’s NeoFace® Reveal [9] is a software solution for forensic investigation that provides law enforcement and crime laboratory agencies with the ability to enhance poor quality latent face images, search them against their mugshot databases, and locate potential suspects, while the NeoFace® Watch integrates with existing video surveillance systems and matches faces in real-time against a watch list of individuals to trigger an alert.
The stated aim is to increase the efficiency of the Surat police.
Tamil Nadu:
The Tamil Nadu Police has started utilizing FaceTagr[10] to reduce crime and increase efficiency.
The police have already made several arrests using this technology.
Legal Status of FRTs in India
The main law that covers this is the Personal Data Protection Bill of 2019[11]. Under Clause 3(7) of the same bill, facial image data comes under the category of “biometric data” along with “fingerprints, iris scans, or any other similar personal data resulting from measurements or technical processing operations carried out on physical, physiological, or behavioural characteristics of a data principal, which allow or confirm the unique identification of that natural person;”
Chapter VIII of the Bill lays out the exemptions where the provisions of the bill do not apply. Clause 35 states that:
“Where the Central Government is satisfied that it is necessary or expedient, —
in the interest of sovereignty and integrity of India, the security of the State, friendly relations with foreign States, public order; or
for preventing incitement to the commission of any cognizable offence relating to sovereignty and integrity of India, the security of the State, friendly relations with foreign States, public order,
it may, by order, for reasons to be recorded in writing, direct that all or any of the provisions of this Act shall not apply to any agency of the Government in respect of the processing of such personal data, as may be specified in the order subject to such procedure, safeguards and oversight mechanism to be followed by the agency, as may be prescribed.”
The clause essentially provides a broad mandate for government agencies to skirt the terms of this bill in the interest of national security, and to mitigate threats against the sovereignty and integrity of the country.
Clause 36 also extends this exemption to when “personal data is processed in the interests of prevention, detection, investigation and prosecution of any offence or any other contravention of any law for the time being in force;”
These two clauses combined, essentially allow the government and its associated agencies to access the personal data of its citizens as and when they decide that a threat to the country exists. This extremely broad mandate has raised concerns with digital rights advocates. In the next section, we will examine these concerns and analyse the case against FRTs.
The Case Against FRTs
There are two aspects to this case:
The legal aspects and challenges of using FRTs.
The technological challenges and flaws of FRTs.
Legal Issues
The major cause for concern is the infringement of the right to privacy. Other than the Personal Data Protection Bill, Indian laws that regulate this technology are scarce. The Information Technology Act (2000) [12] Section 43A provides the conditions for compensation if there was a failure to protect data by a “body corporate”, and Article 72A provides the terms of compensation in the event of improper disclosure of data that is in breach of a lawful contract. The Aadhaar (Targeted Delivery of Financial and Other Subsidies) Act 2016[13], provides some protection as well. Article 30 of the same Act classifies information gathered as “sensitive personal information”, meaning that the provisions provided in the Information Technology Act for the protection of this data apply to information collected under the Aadhaar Act as well.
There have also been several cases and judgements that deal with privacy. The most famous, of course, is the case of Justice K. S. Puttaswamy (Retd.) vs Union of India [14] (hereinafter the Aadhaar Judgement). This landmark judgement by the Supreme Court of India laid out the contours of the right to privacy in India. The judgement established that the right to privacy is a fundamental right. This right includes autonomy over personal decisions (e.g., consumption of beef), bodily integrity (e.g., reproductive rights), as well as the protection of personal information (e.g., the privacy of health records).
The key aspect of this judgement is the proportionality test that was established by the court. The test [16] has three aspects:
The procedure established by law:
The first requirement is that there must be a law in existence to justify an encroachment on privacy (as stated in Article 21).
This means that the infringement must be in accordance with the procedure established by law.
Reasonable classification:
Article 14 of the Indian Constitution mandates a reasonable classification test to guard against arbitrary state action.
What this means is that if the right to privacy is infringed upon or restricted by using the law, that law must fall in the “zone of reasonableness”.
The test of reasonable classification has two criteria:
Classification based on intelligible differentia i.e., there is a clear difference between those in the group and those out of the group.
The classification must be related to the objective of the law or act.
Proportional benefit:
The aims employed need to be proportionate to the benefit sought.
While the AFRS system would be in accordance with the procedure established by law if the Personal Data Protection Bill is passed, without it there exists no anchoring legislation for the AFRS currently.
As per the RFP, “The AFRS will be a centralized web application hosted at the NCRB Data Centre in Delhi with DR in a non-seismic zone which will be made available for access to all the police stations of the country.”
This essentially means that the AFRS would be active all over the country, and every citizen of India would fall under its purview. The AFRS does mention that the intended targets of the system are “criminals, missing children/persons, unidentified dead bodies and unknown traced children/persons.” But as the Supreme Court stated in the Aadhar Judgement, sweeping provisions that target every person in the country cannot be implemented under the guise of preventing crime. As a result, the AFRS system fails the reasonable classification test as well, because there is no differentiation provided.
The system is also slated to have identification capabilities, meaning that the government would have to collect sensitive data and enrol it into the recognition system to make it work. Data collection at this scale would not be proportionate to the slated end objective as it is highly likely that a majority of the data would go unused. Therefore, the system also fails the proportionality criterion of the test.
Technological flaws
To further solidify the case against the AFRS, let us take the steel man approach. Assume that somehow a respondent to the RFP comes up with an approach that does satisfy the aforementioned test laid out in the Aadhar Judgement i.e., the system is legally sound and does not violate anyone’s rights. Are there any more concerns about implementing the AFRS system?
The answer to this question is still a yes. The reason for this is that the entire concept of the AFRS is built on a single assumption: that FRTs are actually accurate. In reality, they are not.
In 2015[16], a story broke regarding Google Photos. The Facial Recognition software that Google Photos used (at that point), labelled some pictures of African-Americans as “Gorillas”. Google worked to quickly rectify the error.
Now, the fact that this incident happened in 2015 might alleviate some concerns. Technology surely must have improved in the six years since. Unfortunately, FRTs are still ridden with inaccuracies [17][18]. A study [19] conducted by MIT concluded that Amazon’s facial recognition software incorrectly determined the sex of female and dark-skinned faces. The researchers called upon Amazon to stop selling such faulty technologies that have been implicated in the misidentification and arrests of innocent people with little or no accountability from the companies involved.
This happens because of two factors:
A small homogenous group of people hold an asymmetric degree of control over the development of the systems and the algorithms that are built into the systems.
No accountability or safeguards when the algorithms incorrectly identify someone as being a criminal etc.
First, let us examine what the asymmetric control over the systems, their development and their algorithms mean. It is a general misconception that algorithms are unbiased. The reality is that algorithms are merely the products of their creators, therefore the bias and poor decision-making of the creators during development becomes apparent during the system's operation in the real world.
For example, take the inability of most present-day FRTs to accurately identify the faces of people of colour. The root cause of this issue is simple: biased training data. Training data is a collection of labelled information that is used to build a machine learning model. It usually consists of annotated text, images, video, or audio. Through training data, an Artificial Intelligence model learns to perform its task at a high level of accuracy.
However, if the training data itself is flawed or biased, the system will replicate those biases. If the facial data fed to the FRTs mostly consisted of white cis-male faces, the system would no doubt only recognise faces falling within this category. Biased training data leads to biased decision-making by the system which means that the biases of those who are compiling this training data will be reflected in these systems.
IBM's facial recognition technology was withdrawn [20] on the grounds of racial bias. In June 2020, IBM announced they would withdraw “general purpose IBM facial recognition or analysis software” citing racial bias among several concerns. In a letter to the U.S. Congress, IBM’s CEO, Arvind Krishna, called for policies to determine whether law enforcement agencies could use such technology “responsibly.” The letter states that following the deaths of George Floyd, Ahmaud Arbery, Breonna Taylor, the fight against systemic racism was far from over. The letter highlights the lack of accountability that comes with this technology, which in conjunction with the already accountability lacking United States Police, would be a recipe for amplifying systemic injustice.
In her testimony [21] to the House Oversight Committee about the regulation of facial recognition technology Joy Buolamwini, founder of the Algorithmic Justice League, pointed out this exact issue. The FRTs have been rapidly expanding with little to no oversight over their development, their effects or their accuracy.
The dangers of this bias are extreme. The technology could become another tool of systemic oppression enabling the state to further punish marginalised peoples. In the same aforementioned testimony, Joy Buolamwini brought up the following case:
“In April of 2019, a Brown University senior and Muslim activist, Amara K. Majeed, was misidentified by facial recognition technology as a terrorist suspect in the Sri Lanka Easter bombings. As a woman of colour under the age of 25, she fit demographic groups (women and youth) that former FBI facial recognition expert and colleagues recorded to be most susceptible to inaccuracies from facial recognition systems they tested in a seminal study on the impact of demographics on the accuracy of facial recognition technology. The police department later issued a statement correcting the error, yet the damage had already been done. According to the Boston Globe, Ms Majeed received death threats as a result of the mistake, her family members in Sri Lanka were exposed to greater police scrutiny, and as a student studying for finals at the time of misidentification, her academic performance was also put at risk.[22]”
Facial Recognition Systems are carceral tools of the state that disproportionately affect those who are underrepresented and amplify systemic differences. Marginalised peoples are more likely to be targeted by these systems due to their biases, and the effects that this system could have are significant. Housing, education and employment [23] are some other areas where this technology has been deployed and we see that it disproportionately affects minorities in these critical areas as well. To understand this better, let us take the case of housing. Landlords may fail to obtain affirmative consent and fail to ensure that the facial recognition system performs accurately for all groups of tenants, including women, children, the elderly and people of colour especially considering the intersectionality of these groups (for example, women of colour).
The technology also has the potential to lend itself to discriminatory hiring practices. Companies have shifted to using FRTs to streamline the recruitment process. Hiring systems are trained on data from top performers. However, if the data favours one group based on gender or race, then the system will generate recommendations in favour of the group in providing recommendations for hiring.
Another example is a report [24] on Project Green Light (PGL), which was enacted in 2016, and consisted of installing high-definition cameras throughout the city of Detroit found that “surveillance and data collection was deeply connected to diversion of public benefits, insecure housing, loss of employment opportunities, and the policing and subsequent criminalization of the community members that come into contact with these surveillance systems”.
The second major area of contention with such systems is a lack of accountability and oversight on the part of both the users of this technology and the makers of it. There are two parts to this:
Lack of accountability or standards regarding the collection of facial data.
Lack of accountability or standards when the system fails to reach the correct outcome.
As mentioned previously, Indian legislation about the collection of data is inadequate and India's situation is not unique. At the federal level in the United States, there is some legislation such as the Privacy Act of 1974, which limits the ability of federal agencies to collect data but it does not apply to States or private companies [25]. States must pass their consumer data protection legislation and only three states (California, Colorado and Nevada) have done so. The rest are yet to catch up [26]. In 2011, Facebook automatically enrolled all of its users in its face recognition program without obtaining consent [27]. The United Kingdom also has a similar system that has raised concerns.[28]
When the algorithm makes a decision and incorrectly identifies someone as a criminal, terrorist or as high-risk, there is no transparency as to how the system came to this decision. The assumption is that as it is a computer making the decision, the decision is therefore accurate. However, as we have seen, computers are merely tools of those who program them to achieve them and thus the biases of the creators may be replicated in the creation.
However, due to the assumption that these systems are infallible there are few (if any) redressal mechanisms for those who are wrongfully targeted, as our legal system has not yet caught up with technology. The systems are also not transparent, and how they make decisions and how they are developed is not known.
Due to these reasons, even if the systems somehow were legally sound, the problems with their accuracy would lead to wrong or unjust outcomes.
Recommendations
So, what now? What are the steps that governments need to take to ensure the correct use of this technology? There are a few that Joy Buolamwini highlights in her testimony. These steps are:
Moratorium on face surveillance technology until there are legal limitations and protections.
The State of California in 2018 enacted a bill [29] regarding a 3-year moratorium prohibiting the use of FRTs in police body cameras.
Existing systems need to take affirmative consent from the user i.e., allow users to opt-in to the technology.
Under the General Data Protection Regulation (GDPR) [30], face data is classified as sensitive personal data.
As a result, explicit consent must be given by an individual before their data is collected.
Addressing bias:
Require vendors to implement internal bias evaluation, mitigation and reporting procedure, and support third-party verification/independent evaluation from the research community.
Report compliance with national benchmarks where available.
Decriminalise beneficial research that could allow for improvements in these systems.
Addressing transparency
Institute transparency requirements i.e., customers and users need to know when and how the data is being used in consumer products and services, and have a choice in whether or not their face data is captured, stored, sold and/or used to enhance the technical capabilities of the vendor or third parties.
Mandate disclosure of use when the technology is used in areas such as housing, health, education etc.
Conclusion
It is important that when we are discussing the utilisation of new technologies, we analyse their effects on society. Technology is not always an unbiased tool and a tool for good. We must therefore remain vigilant towards possible instances of function creep (the gradual widening of the use of a technology or system beyond the purpose for which it was originally intended, especially when this leads to potential invasion of privacy) under the guise of making our lives better. Developers must also be sensitised to these issues when developing these tools, preferably making courses that deal with sociology and ethics a mandatory part of computer science programs taught around the world.
References
[1]: National Crime Records Bureau (NCRB), Ministry of Home Affairs, Government of India. (2020, June 22). Request For Proposal To procure National Automated Facial Recognition System (AFRS). Retrieved from: https://ncrb.gov.in/sites/default/files/tender/AFRSRFPDate22062020UploadedVersion.pdf
[2]: Buolamwini, J., Ordóñez, V., Morgenstern, J., & Learned-Miller, E. (2020, May). FACIAL RECOGNITION TECHNOLOGIES: A PRIMER. https://global-uploads.webflow.com/5e027ca188c99e3515b404b7/5ed1002058516c11edc66a14_FRTsPrimerMay2020.pdf
[3]: M. Ehrlich, T. J. Shields, T. Almaev and M. R. Amer, "Facial Attributes Classification Using Multi-task Representation Learning," 2016 IEEE Conference on Computer Vision and Pattern Recognition Workshops (CVPRW), 2016, pp. 752-760, doi: 10.1109/CVPRW.2016.99.
[4]: Kaspersky. (2021, April 26). What is Facial Recognition – Definition and Explanation. https://www.kaspersky.com/resource-center/definitions/what-is-facial-recognition
[5]: Project panoptic. (2020). Panoptic. https://panoptic.in/
[6]: Government of Telangana. TSCOP. https://tweb.telangana.gov.in/departments/home-department/telangana-state-police/tscop/
[7]: Government of Telangana. CCTNS.
[8]: Mengle, G. S. (2019, July 29). Mumbai Police get first-of-its-kind biometric criminal tracking system. The Hindu. https://www.thehindu.com/news/cities/mumbai/mumbai-police-get-first-of-its-kind-biometric-criminal-tracking-system/article28752819.ece
[9]: NEC. (2020, February 25). NEC provides Face Recognition Technology to Surat City Police [Press release]. https://www.nec.com/en/press/201502/global_20150225_03.html
[10]: Facetagr – Face Recognition. Video Analytics. https://facetagr.com/
[11]: Personal Data Protection Bill, Bill No. 373, Lok Sabha(2019), http://164.100.47.4/BillsTexts/LSBillTexts/Asintroduced/373_2019_LS_Eng.pdf
[12]: THE INFORMATION TECHNOLOGY ACT (2000), Act No. 21, Lok Sabha(2000), https://www.indiacode.nic.in/bitstream/123456789/1999/3/A2000-21.pdf
[13]: THE AADHAAR (TARGETED DELIVERY OF FINANCIAL AND OTHER SUBSIDIES, BENEFITS AND SERVICES) ACT, Act No. 18, Lok Sabha(2016), https://uidai.gov.in/images/targeted_delivery_of_financial_and_other_subsidies_benefits_and_services_13072016.pdf
[14]: Justice K.S.Puttaswamy(Retd) vs Union Of India, Supreme Court of India, 26 September 2018, https://indiankanoon.org/doc/127517806/
[15]: Ibid, para 310, Judgment by Dr. D.Y. Chandrachud, J.
[16]: Zhang, M. (2015, July 1). Google Photos Tags Two African-Americans As Gorillas Through Facial Recognition Software. Forbes. https://www.forbes.com/sites/mzhang/2015/07/01/google-photos-tags-two-african-americans-as-gorillas-through-facial-recognition-software/?sh=108a466a713d
[17]: Hill, K. (2020, August 3). Wrongfully Accused by an Algorithm. The New York Times. https://www.nytimes.com/2020/06/24/technology/facial-recognition-arrest.html
[18]: A Black man spent 10 days in jail after he was misidentified by facial recognition, a new lawsuit says. (2020, December 30). Business Insider. https://www.businessinsider.com/black-man-facial-recognition-technology-crime-2020-12?r=DE&IR=T
[19]: Raji, I & Buolamwini, J. (2019). Actionable Auditing: Investigating the Impact of Publicly Naming Biased Performance Results of Commercial AI Products. Conference on Artificial Intelligence, Ethics, and Society.
[20]: Krishna, A. (2020, November 9). IBM CEO’s Letter to Congress on Racial Justice Reform. IBM. https://www.ibm.com/blogs/policy/facial-recognition-sunset-racial-justice-reforms/
[21]: Facial Recognition Technology (Part 1): Its Impact on our Civil Rights and Liberties, Hearing before the United States House Committee on Oversight and Government Reform, 116th Cong. (2019) (Written testimony of Joy Buolamwini). https://docs.house.gov/meetings/GO/GO00/20190522/109521/HHRG-116-GO00-Wstate-BuolamwiniJ-20190522.pdf
[22]Cox, J. C. (2019, April 28). Brown University student mistakenly identified as Sri Lanka bombing suspect. The Boston Globe. https://www.bostonglobe.com/metro/2019/04/28/brown-student-mistaken-identified-sri-lanka-bombings-suspect/0hP2YwyYi4qrCEdxKZCpZM/story.html
[23]: Algorithmic Justice League. (n.d.). What is facial recognition technology? Retrieved June 21, 2021, from https://www.ajl.org/facial-recognition-technology
[24]: Detroit Community Technology Project. (2019, June). A Critical Summary of Detroit’s Project Green Light and its Greater Context. https://detroitcommunitytech.org/system/tdf/librarypdfs/DCTP_PGL_Report.pdf
[25]: The Privacy Act of 1974, Pub Law No. 93-579, 88 Stat 1896 (Dec. 31, 1974), 5 U.S.C. § 552a (2018). https://www.govinfo.gov/content/pkg/USCODE-2018-title5/pdf/USCODE-2018-title5-partI-chap5-subchapII-sec552a.pdf
[26]: International Association of Privacy Professionals. (2021, June 11). US State Privacy Legislation Tracker. https://iapp.org/resources/article/us-state-privacy-legislation-tracker/
[27]: What Facial Recognition Technology Means for Privacy and Civil Liberties, Hearing before the Senate Committee on the JudiciarySubcommittee on Privacy, Technology, and the Law, 112th Cong. (2012) (Written Testimony of Jennifer Lynch). https://www.judiciary.senate.gov/imo/media/doc/12-7-18LynchTestimony.pdf
[28]: Burton, L. (2021, March 31). Facial recognition technology: police powers and the protection of privacy. House of Lords Library. https://lordslibrary.parliament.uk/facial-recognition-technology-police-powers-and-the-protection-of-privacy/
[29]: California State Legislature. (2019, October 8). Bill Text - AB-1215 Law enforcement: facial recognition and other biometric surveillance. https://leginfo.legislature.ca.gov/faces/billNavClient.xhtml?bill_id=201920200AB1215
[30]: REGULATION (EU) 2016/679, on the protection of natural persons with regard to the processing of personal data and on the free movement of such data, and repealing Directive 95/46/EC (General Data Protection Regulation), THE EUROPEAN PARLIAMENT AND OF THE COUNCIL, https://eur-lex.europa.eu/legal-content/EN/TXT/PDF/?uri=CELEX:32016R0679&from=EN